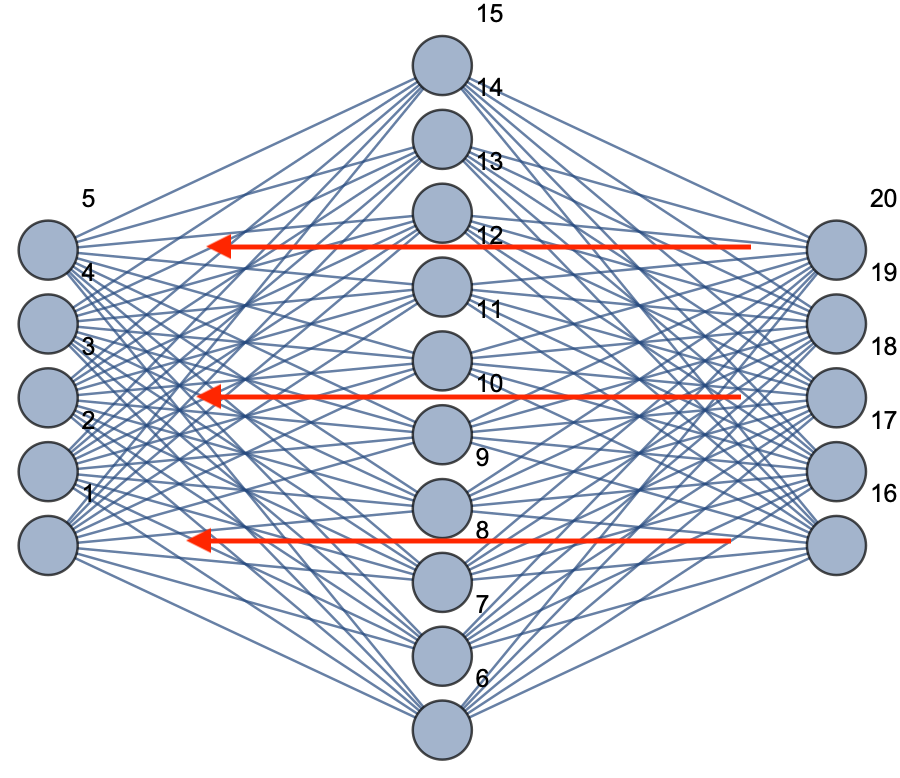
人工神经网络的反向传播-梯度下降算法
关于人工神经网络 人工神经网络模型判断手写数字 我们首先在Mathematica中徒手打造一个人工神经网络,然后对该神经网络进行训练,最后用训练得到的模型来判断图片上的手写数字是多少。 trainingData = ResourceData["MNIST", "TrainingData"]; testData = ResourceData["MNIST", "TestData"]; net = NetInitialize@NetChain[{ FlattenLayer[], LinearLayer[500], ElementwiseLayer["Sigmoid"], LinearLayer[100], ElementwiseLayer["Sigmoid"], LinearLayer[10], SoftmaxLayer[]}, "Input" -> NetEncoder[{"Image", {12, 12}, "ColorSpace" -> "Grayscale"}], "Output" -> NetDecoder[{"Class", Range[0, 9]}]] NetEncoder告诉我们输入的单通道12*12的图像 NetDecoder告诉我们我们的神经网络模型最后输出的是一个分类,类别分别是0-9中的一个数字 网络层的模型为: FlattenLayer[] 图像中的像素数据即两纬数据排列成一纬数据做为输入 LinearLayer[500] 第一层线性层,输出数据为500个,限定函数为Sigmoid LinearLayer[100] 第二层线性层,输出数据为100个,限定函数为Sigmoid LinearLayer[10] 最后一层输出,因为我们需要有10个分类,所以这里设定是10,限定函数为 Softmax 神经网络层形象的展示如下: 训练数据标记,左边是实际的手写数字,右边是对应标签 RandomSample[trainingData, 10] 在未对模型进行训练前,使用随机的权重来判断图片,我们可以确定错误率接近100% 对模型进行训练 trained = NetTrain[net, trainingData, ValidationSet -> testData, MaxTrainingRounds -> 50 ] 从以上图表中我们大致可以看出正确率已经接近97% 再次预测上述图片,模型已经可以正确预测给出的图片数字为6 measurements = ClassifierMeasurements[trained, testData] 运行 measurements[“Accuracy”] 我们可以得到模型的正确率为98.02% ...